Swarm Learning: harnessing AI power for better medical care

The Systems Medicine Department at the German Center for Neurodegenerative Diseases (DZNE) has achieved a groundbreaking milestone with the launch of the DZNE Swarm Learning Hub. This cutting-edge initiative marks a transformative step in leveraging decentralized artificial intelligence to revolutionize medical research. By integrating Sswarm Llearning — a collaborative AI approach that securely pools insights from multiple locations without sharing sensitive data — the hub promises to unlock unprecedented opportunities for understanding complex diseases. This innovation underscores the DZNE's commitment to pioneering advanced technologies that foster data-driven breakthroughs in healthcare.
In an exciting development, the DZNE Swarm Learning Hub has been chosen to host the Competence Hub for Swarm Learning of ImmunoSensation³, a prestigious the Cluster of Excellence dedicated to deciphering the immune system. This partnership unites leading minds in immunology and AI to accelerate discoveries in immune health, aging, and disease prevention. By combining expertise in cutting-edge computational methods with world-class immunological research, this initiative aims to set new benchmarks for interdisciplinary collaboration. Together, they are poised to redefine the future of medicine, creating a robust foundation for innovations that will improve lives across the globe.
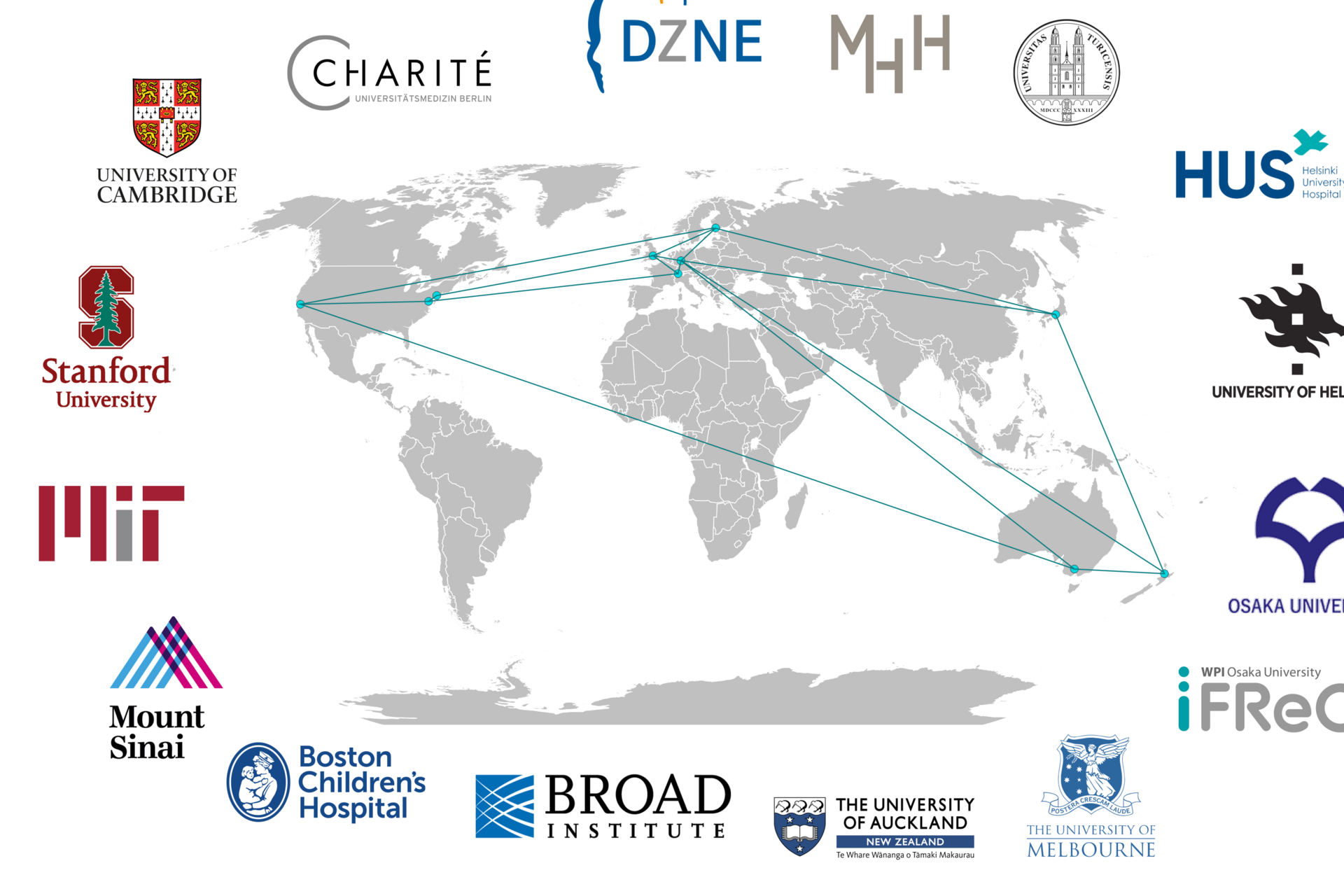
Identifying patients with critical diseases is a key objective of precision medicine. Molecular characterization of patient biosamples using 'omics' technologies has opened avenues for the use of large-scale data for diagnostic purposes, especially including the application of artificial intelligence (AI)-based pattern recognition. In global crises, reliable, fast, secure, and privacy-preserving AI solutions can help address crucial questions in combating these threats.
AI solutions depend on appropriate algorithms and, more importantly, large training datasets. Due to the decentralized nature of medicine, local data volumes are often inadequate for training reliable and generalizable classifiers. To implement a technical solution avoiding unnecessary data traffic and duplication as well as honoring European privacy protection laws, we initiated the Global Swarm Learning Project connecting institutions around the world.
Profiling the blood immune cells by high-dimensional cytometry is one of the fundamental approaches in immunology to phenotype the status of the immune system of the donor and thereby their health status. In the Global Swarm Network, we are now harmonizing, standardizing and optimizing the approaches to use high-dimensional cytometry for AI-based analysis in a joint fashion around the globe.
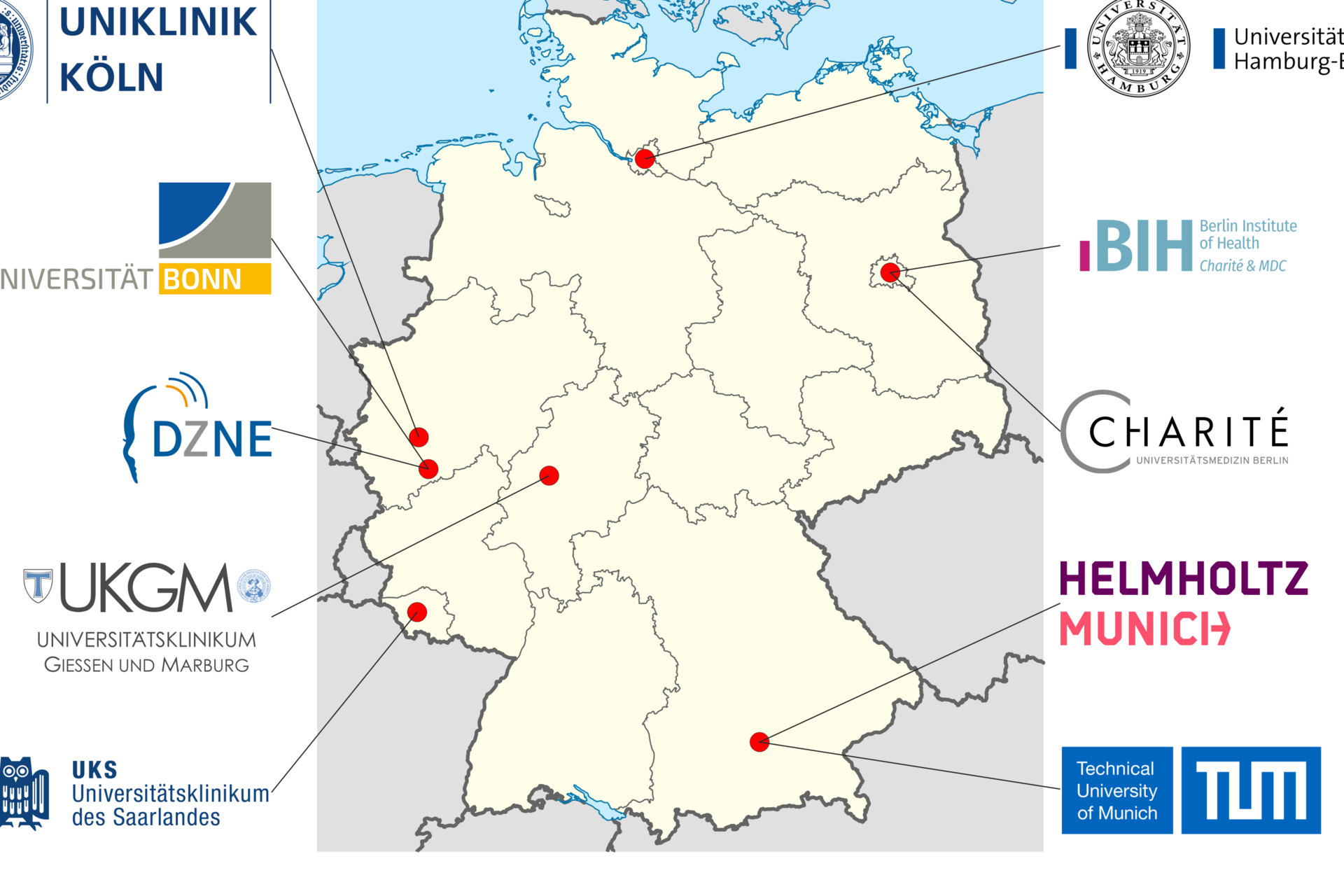
Medicine and research are generating an increasing amount of data, leading to a more detailed understanding of diseases and their underlying processes. In this complex landscape of information, there is potential to discover essential insights for developing new precision therapies that can be better tailored to individual patients than conventional treatments.
In this project, funded by the Volkswagen Foundation, experts from Bonn, Berlin, Cologne, Giessen, Hamburg, Homburg, Munich, and Saarbrücken are connecting clinics and institutions across Germany in a national Swarm Learning network. As a case study, COVID breakthrough infections are studied to understand why some people contract COVID despite being vaccinated.
The project also focuses on ethical aspects of the use of AI in medicine. Engagement with patient support groups as well as experts with ethical expertise will ensure addressing ethical and social considerations during the project and not only after implementation of technical innovations, as is unfortunately often the case.
In global crises, reliable, fast, secure, and privacy-preserving AI solutions can help address crucial questions in combating these threats.
Members of the Consortium
- Prof. Dr. Marylyn Addo, University Medical Center Hamburg-Eppendorf
- Prof. Dr. Alena Buyx, Technichal University Munich
- Prof. Dr. Susanne Herold, University Hospital Giessen and Marburg
- Prof. Dr. Florian Klein, University Hospital of Cologne
- Prof. Dr. Percy Knolle, Technical University Munich
- Prof. Dr. Leif Erik Sander, Charité - Universitätsmedizin Berlin, Co-Speaker
- Prof. Dr. Birgit Sawitzki, Charité - Universitätsmedizin Berlin
- Prof. Dr. Martina Sester, Saarland University Hospital
- Prof. Dr. Joachim Schultze, Deutsches Zentrum für Neurodegenerative Erkrankungen (DZNE) and University of Bonn, Speaker
- Prof. Dr. Fabian Theis, Helmholtz Munich
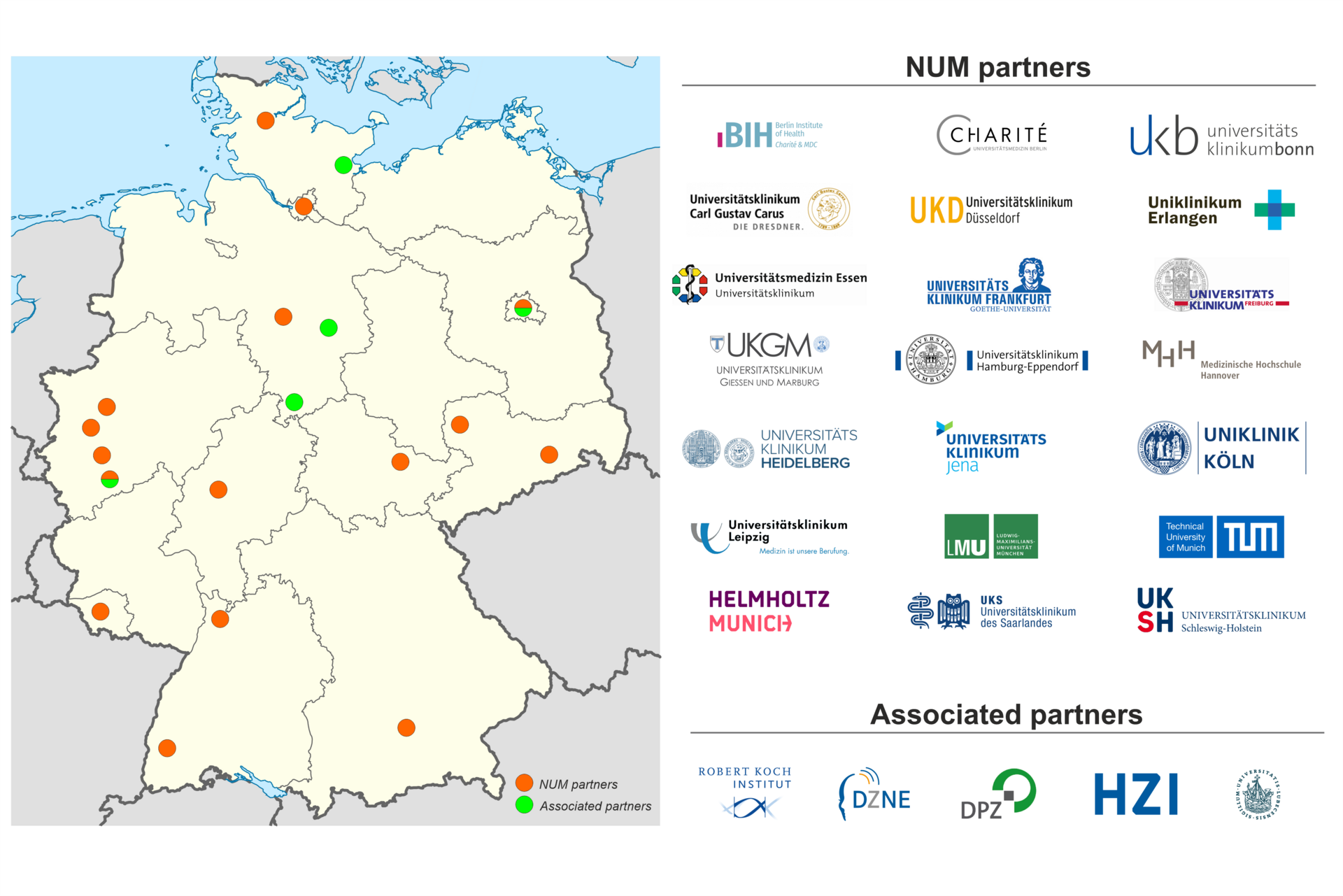
COVIM (Collaborative Immunity Platform of the NUM) is a nationwide network of leading scientists and clinicians specializing in immunology, virology, clinical infectious diseases, epidemiology, and data science.
During its initial phase of funding, the COVIM consortium examined immune responses to SARS-CoV-2 induced by infection and vaccination, aiming to define the extent and duration of immunity against COVID-19. Essentially, the goal was to determine who is protected against COVID-19, when, and for how long.
To assess population immunity during a pandemic and identify at-risk groups, validated and standardized procedures for measuring immunity are essential, alongside appropriate clinical studies and cohorts. A thorough understanding of the immune response to SARS-CoV-2 also facilitates the development of new prophylactic and therapeutic treatments for COVID-19 based on the principles of protective immunity.
In its second phase of funding, COVIM will establish and expand scalable infrastructures for the rapid collection and analysis of complex datasets related to immunity against COVID-19 and future pandemic threats. This will enable immediate access to information on the state of immunity against pathogenic threats, allowing for swift responses and scientifically informed decisions regarding potential interventions during outbreaks.
The overall project is an integral part of the Network of University Medicine (NUM) and is closely integrated with NUM infrastructures and other research projects.

The DZNE is connecting the different DZNE sites with Swarm Learning capabilities. This will allow the DZNE to run joined analyses in research projects performed at different DZNE sites throughout Germany. Particularly for large data like imaging data, this makes it easier to collaborate without the need to exchange large raw data between different sites or move data to a central data repository.
Another future use of Swarm Learning is foreseen within the Clinical Trial Unit network of the DZNE. Here Swarm Learning will support multi-center clinical trials performed at the different DZNE sites within the Clinical Trial Units.